How AI can help you integrate a new team faster (Part 2).
Copy/paste this prompt to instantly generate a market history analysis report.
Thank you for reading The Product Courier!
We’re 1,891 here, which is 248 more than last month! 🚀
If you're new here, welcome! I’m Lucas Nilsson, a Product Marketing freelancer helping early-stage startups refine their positioning, messaging and pricing to go to market effectively.
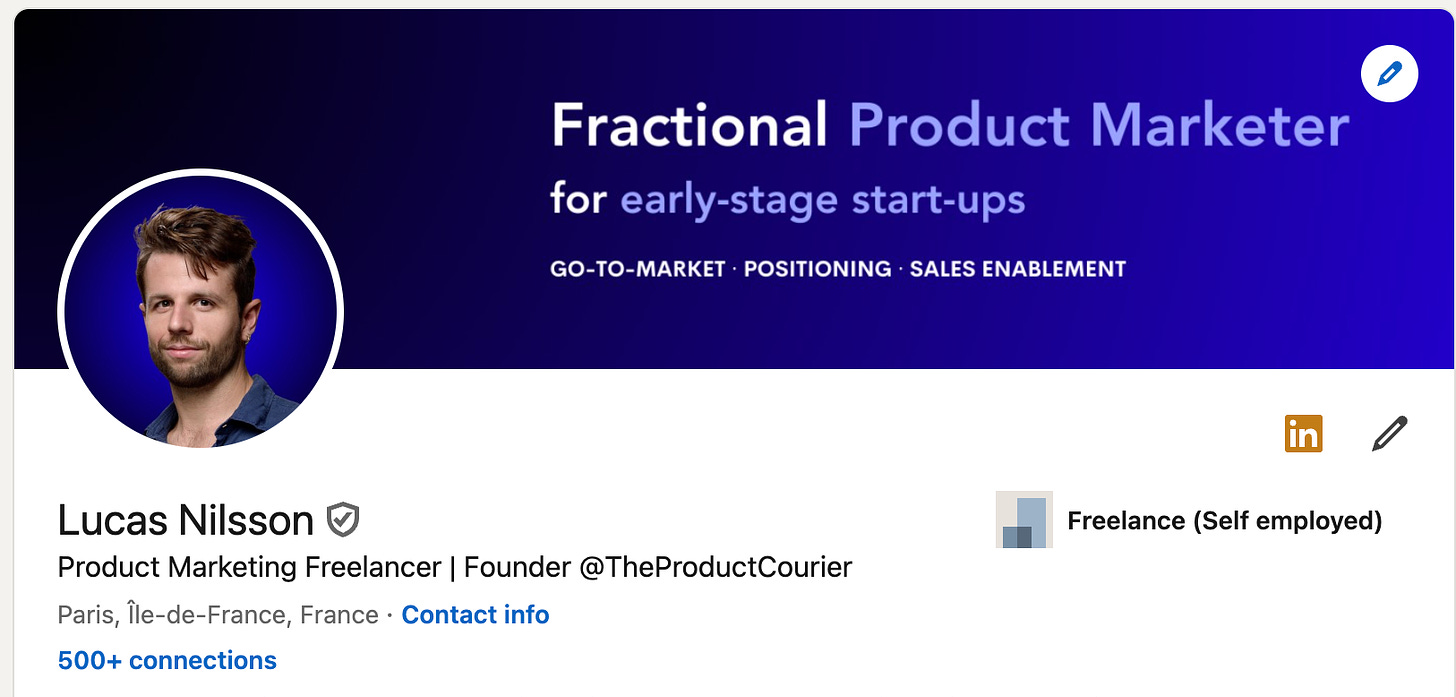
Together with my partner Toni Dos Santos, we highlight each week 1 AI use cases for PMs and PMMs. Our goal is to show you how AI can enhance your daily job.
In this 33rd edition, we’ll talk about:
Why scaling is the key to the future of AI. 📰
Your weekly use cases: Integrate a new team faster with AI (Part 2). 🔍.
Make sure you subscribe to not miss our next tutorials!
News of the week. 📰
AI progress is slowing down. Scaling to the rescue?
Whether AI is just hype or not is still up for debate.
I think right now we're in a phase of unrealistic expectations around AI. We’ve gotten used to big breakthroughs every six months and tech gurus telling us we’re going to reach AGI (Artificial General Intelligence—AI that can learn and do anything a human can) within a year.
At this point, though, that feels pretty unlikely.
That said, GenAI technologies are already incredibly powerful, and we’re nowhere near using their full potential, so calling it all "hype" seems a bit off.
AI innovation does seem to be slowing down, mainly because companies are running into some big challenges, like:
Not enough data
Limited access to computing power
Model size limitations
Dario Amodei, the CEO of Anthropic, talked about this recently in a five-hour (!) interview with Lex Fridman. A lot of the conversation was about scaling theory—the idea that if we just had more data, more compute, and bigger models, AI would keep getting better.
II think this is a great chance to bring up these challenges. We’ll probably dive deeper into each one in the upcoming editions.
More Data + More Compute + Bigger Models = Better AI?
Progress in the capabilities of AI technologies is predictably driven by progress in three inputs—compute, data, and algorithms.
Each of these are now facing challenges:
Lack of data is a big problem because AI models need MASSIVE quantity of great quality and diverse data. Without enough data or data that reflects the real world accurately, these models fall short of expectations.
👉 One solution could be synthetic data generation. Synthetic data are data that are generated by AI itself. They can help create diverse datasets that are still useful for training AI models, reducing the dependency on real-world data.Access to computing power is another issue. Training advanced AI models is very expensive – ChatGPT was reportedly costing $700,000 dollars per day in compute power. Big companies like OpenAI and Google have the resources for this, but many smaller innovators do not, which creates a gap and slows progress.
👉 A possible solution would be to develop more efficient algorithms that require less computing power. Additionally, investing in specialized hardware, like specialised AI chips, could help cut costs and reduce energy consumption, making AI development more accessible to start-ups.Model size is also a challenge. There has been a push to create bigger models with billions of parameters, under the idea that bigger is better. But larger models are harder to use, need more computing power, and are harder to understand. Many now believe that instead of making models bigger, they should be made more efficient, easier to understand, and specialized for specific tasks.
👉 One way to address this is to optimize models for specific tasks, making them smaller and more efficient without losing performance.
Basically, the future of AI might look like this: more specialized models, trained on a mix of human and AI-generated data, running on cost-effective, specialized AI chips.
However, key figures in the AI sector such as Ilya Sutskever (former Chief Scientist of OpenAI), doubts that scaling will solve the current challenges that the industry is facing.
Community Polls. 📊
Your weekly tutorial. 🔍
Analyze any market history in < 10 seconds.
TL;DR: ✅
In the previous edition of this series on research, I shared my strategy for quickly getting up to speed with any product team using AI.
After focusing on understanding the technology and terminology, this edition dives into how to gather market history insights.
With the prompt we've created, you can generate a full report that gives you the key insights you need about an industry.
You can also use these results to create or enrich your knowledge base or include them in your internal training materials.
Analyze the past to understand the present.
"The more you know about the past, the better prepared you are for the future."
— Theodore Roosevelt
If you want to succeed as a product professional, understanding the history of your companies market is key.
It helps you see how technology has changed over time and why things are the way they are today. This kind of knowledge gives you the context you need to make better decisions going forward.
In the past, I used to sit down with developers or product managers and ask them to explain the technology and its history. I’d ask a lot of questions to try and make sense of their work and the products they were building.
I still do this, but now I focus more on the finer details and understanding customers.
Thanks to AI, I can get a general understanding of the technology on my own. I then use conversations with the experts to dig deeper into the specific parts that matter most.
AI is especially helpful when it comes to understanding market history. It can quickly put together a simple report that sums up everything you need to know.
In just a few seconds, you can get a complete picture that includes:
Key technological advancements that have shaped the industry.
Important changes in regulations that have influenced the market.
Shifts in customer needs and preferences, showing how they’ve changed over time.
Current market trends and where the industry is heading.
It’s not just about knowing what’s happening now—it’s about understanding how we got here and what might happen next.
In Part 1 of this series, we talked about how to quickly understand a technology and its users.
Now, in Part 2, we’ll look at how you can use AI to create a market history report with the prompt we created.
Market history report:
Example with the Flight Comparator industry 👇
1. Use case:
For this demonstration, we’re going to use the following scenario.
I’m a new Product Marketing Manager joining the Google Flights team.
My primary product is a flight comparison tool—designed to help users efficiently find and compare flight options across airlines.
I want to get a quick overview of the history of the market and its main trends in order to rapidly understand the main market dynamics and accelerate my onboarding in my new position.
2. Prompt.
(👉 Subscribe to The Product Courier Premium to get access to this prompt and 27 others).
3. Results. ✨
Here’s the output I got from ChatGPT after copy/pasting this prompt. 👇
Flight Comparator: A comprehensive history analysis
The purpose of this report it is to provide a comprehensive overview of the industry's growth trajectory, identifying pivotal moments and trends that continue to define its landscape.
1. Timeline of Key Events and Milestones
Late 1990s to Early 2000s: Birth of Online Travel Agencies (OTAs)
The emergence of online travel agencies such as Expedia (1996) and Priceline (1997) marked the beginning of a major shift in the travel industry. These platforms made it possible for consumers to book flights online for the first time, paving the way for future aggregators.
2003-2007: Emergence of Flight Comparison Platforms
Kayak, founded in 2004, was among the first dedicated flight comparators to focus specifically on aggregating flight data from multiple sources. This period saw other players, such as Skyscanner (2001) and Momondo (2006), enter the market, establishing the foundation for a growing industry.
2010-2015: Expansion and Industry Consolidation
During this time, the industry began to see significant consolidation, with larger companies acquiring smaller competitors to expand their market reach. Priceline Group acquired Kayak in 2012, and Ctrip (now Trip.com Group) acquired Skyscanner in 2016. The development of mobile apps also enabled more convenient access for consumers, further driving industry growth.
2018-Present: Rise of AI and Machine Learning
The use of artificial intelligence and machine learning technologies became prevalent, enabling personalized search results, predictive pricing, and enhanced user experiences. Platforms such as Hopper leveraged these technologies to differentiate themselves, providing consumers with price predictions and recommendations on the optimal times to book.
2. Technological Advancements
The flight comparator industry has evolved in tandem with advancements in internet technology, mobile computing, and artificial intelligence. Early platforms were reliant on simple web crawlers to aggregate flight data, but the development of sophisticated APIs has allowed for real-time data integration.
API Integration: APIs became a pivotal technology that enabled platforms to access live data from airlines and OTAs. This development ensured that flight information was current and accurate, improving consumer trust.
Mobile Accessibility: The proliferation of smartphones significantly changed the flight comparator landscape. Mobile apps became a key channel for consumer engagement, with companies optimizing their platforms for mobile users. This shift not only made flight comparison more accessible but also allowed for the introduction of push notifications, alerting users to price changes.
AI and Predictive Analytics: More recently, machine learning has played a central role in improving the accuracy of fare predictions and personalizing recommendations. Companies like Hopper have capitalized on predictive analytics to provide insights into the best times for booking flights, adding value beyond simple price comparison.
3. Regulatory Changes
The flight comparator industry has also been influenced by regulatory changes, particularly those aimed at increasing transparency and consumer protection.
EU Regulation 1008/2008: This regulation mandated that airline pricing be transparent, including all unavoidable taxes and fees in the displayed price. This change made it easier for consumers to compare flights, benefiting flight comparison platforms by enhancing their value proposition.
GDPR (2018): The General Data Protection Regulation impacted how flight comparator websites collected and processed user data. Compliance with GDPR necessitated improvements in data security and transparency, which affected the personalization features offered by these platforms.
Airline Deregulation: Airline deregulation in many countries, particularly in the United States and Europe, allowed for increased competition among carriers, leading to more varied pricing strategies and thus greater demand for flight comparison services.
4. Market Dynamics: Entries, Exits, and Consolidation
The flight comparator industry has seen significant shifts in market dynamics, marked by both the entry of new players and industry consolidation through mergers and acquisitions.
New Market Entrants: The late 2000s and early 2010s saw an influx of new entrants seeking to capitalize on growing consumer interest in comparing travel options online. Startups like Hipmunk (2010) introduced unique user interfaces that differentiated them in a crowded market.
Consolidation Trends: Larger players have acquired many of these new entrants over time. For instance, Priceline's acquisition of Kayak and Ctrip's acquisition of Skyscanner are indicative of an industry that has steadily consolidated to enhance market power and expand consumer reach.
Exit of Players: Not all market players have survived the competition. Hipmunk, despite its innovative approach, was eventually shut down in 2020 after being acquired by SAP Concur in 2016. The inability to achieve profitability or scale sufficiently in a competitive landscape often led to the exit of smaller players.
5. Shifts in Consumer Demand
Consumer behavior has also evolved significantly over the years, influencing the development of flight comparators.
Price Sensitivity and Transparency: From the outset, consumers have been highly price-sensitive, seeking platforms that could provide the lowest fares. The demand for transparency has also increased, leading flight comparators to provide detailed breakdowns of pricing, including additional fees and taxes.
Demand for Personalized Experiences: With the advent of AI, consumer expectations have shifted towards more personalized recommendations. The introduction of predictive pricing, such as the price prediction feature by Hopper, reflects the industry's response to a growing desire for actionable insights, rather than just static comparisons.
Mobile Usage: The rise in mobile device usage has influenced consumer expectations regarding ease of access and convenience. Consumers now expect to be able to compare and book flights from their smartphones, leading to an emphasis on mobile-first design and user experience.
Conclusion: Evolution and Future Prospects
The flight comparator industry has come a long way from the early days of basic aggregation. Technological advancements such as API integration, mobile accessibility, and AI-driven personalization have fundamentally transformed the sector. Regulatory changes have fostered greater transparency, benefiting both consumers and platforms. Market dynamics reveal a trend towards consolidation, while shifts in consumer behavior continue to push the boundaries of innovation within the industry.
Looking ahead, the use of AI and machine learning is likely to continue shaping the industry, particularly in areas like dynamic pricing and enhanced user personalization. Moreover, the integration of sustainable travel options in response to changing consumer priorities regarding environmental impact could be a significant future trend, further evolving the industry landscape.
See? With a single prompt you can easily get a recap of the main events and trends that have shaped an industry. You can now ask ChatGPT follow-up questions to dive on specific area.
💡 You can also use the result of this report to enrich your company knowledge center. I usually use this kind of report to create my sales training for example.
Copy/paste this prompt to instantly generate a market report. ⬇️
(Copy/paste this prompt to get similar results with your own product).
[This section is exclusive to paid subscribers. ❤️]
Here's what you gain when you subscribe to The Product Courier. 👇
Get unlimited access to our 27 prompts library (for annual subscribers only).
Receive 4x articles and tutorials each month.
Access 1 Members-only Q&A session each quarter with us.
Network with our exclusive community of premium users.
Be a board advisor on the future content in The Product Courier.
Keep reading with a 7-day free trial
Subscribe to The Product Courier to keep reading this post and get 7 days of free access to the full post archives.